Artificial Intelligence (AI) is now establishing itself as an essential strategic component for products. Yet integrating it remains a tricky challenge for Product Designers. So how do you turn AI into a real innovation lever, without becoming a gimmick? Feedback from Designers at Cardiologs, Doctrine and Indy!
Remember your first experiences with chatbots... How many made us lose patience by imposing themselves on us with no alternative solution, to the point of interrupting our journey? As a counter-example, let's cite the Shuffle, iconic music player from Apple. As soon as it was launched in 2005, a random mode was natively integrated: its users noticed that the same music came back regularly. The algorithm was therefore redesigned to be less random and offer a better listening experience. AI, yes, but carefully designed!
These two situations reveal that AI integration is a delicate art, governed by good design practice that must be known in order to offer real added value to users.
🤖 Making the Choice of Artificial Intelligence
Is AI really necessary?
Going headlong into a technical solution, without questioning its relevance, conjures up uncomfortable situations for you? Too bad, it's likely to happen again with AI... Indeed, the temptation to solve all problems by this means is great, recalling Maslow's hammer metaphor according to which every problem is a nail for those who have a hammer. From then on, for anyone wishing to use AI, every problem would be data to be processed by an algorithm.
What if, to naturally integrate AI into one's product, the first point was to identify the problem to be solved? Of course, this implies that the problem has been clearly identified. Julien Laureau, Head of Design at Indy, a French start-up marketing accounting software designed for the self-employed and SMEs, insists on this point: "We never do things to follow a trend (...) We always come back to user needs." To achieve this, his team follows a three-stage discovery approach:
- Business issues are identified and explored in advance.
- The problem is carefully reformulated.
- The solution phase generates initial leads. The challenge is to identify solutions and then see if AI can support them.
This is how the use of OCR (Optical Character Recognition) - a technology that offers the possibility of converting images of handwritten or printed text into digital text - allows freelancers to save time by sparing themselves manual input of their expense reports.
Same principle at Cardiologs, a company specializing in digital health, which uses AI to analyze electrocardiograms to detect possible heart abnormalities. Its Lead Designer, Ingrid Pais de Oliveira, insists on the roadmapping process, shared by the Product and Data teams: "The Data Scientists identify something. If the Designers validate it with verbatim, it becomes a roadmap topic."
In both these organizations, no AI is possible without feedback from users, who validate the problem and then the solution. The knowledge that Designers have of users thanks to research therefore plays a fundamental role, enabling:
- Identify concrete use cases such as transaction categorization at Indy, for example.
- Confirm users' appetite for the functionality through interviews and testing.
- To better adapt the algorithm to users, in particular to limit algorithmic bias if necessary.
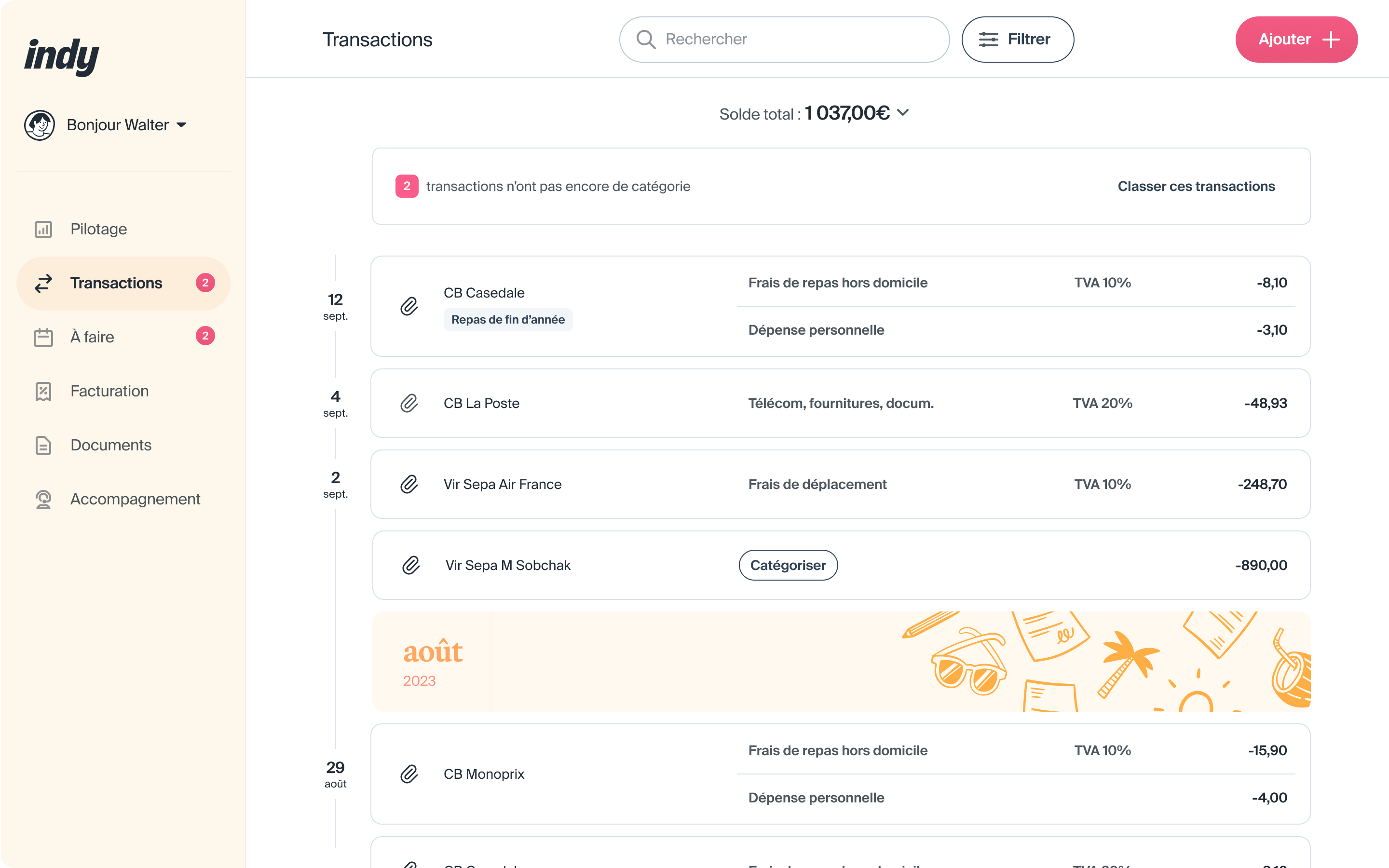
On this last point, let's mention the struggle of Joy Buolamwini, MIT computer science researcher and American-Ghanaian digital activist. She denounces the racial and gender biases in facial recognition algorithms, highlighting their lack of accuracy for the faces of women and racialized people, who were given too little consideration in the testing phases.
Differentiating with AI
When trying to stick perfectly to user expectations, don't we risk missing the ONE good AI use?
This is a major concern for Doctrine, a start-up whose mission is to simplify access to the law, enabling lawyers and jurists to find relevant legal documents thanks to their AI-based algorithms. Its Head of Design, Louis-Éric Maucout, confirms: "We're going to be looking for the differentiating functionality for legal software: what will make the customer say: 'I've never seen this anywhere else'."
Thus, the Doctrine teams have released features eagerly awaited by its users, such as automatic summaries of legal documents or a specialized legal chatbot, but always making sure to add an element of differentiation. In particular, Doctrine stands out by centralizing several legal expertises in a single chatbot. These ideas come in particular from benchmarks fed continuously by all Product, Data and Design teams.
Scenarize AI into existing customer journeys
Once the need has been validated, how do you design the AI solution and integrate it harmoniously into the journey? On this subject, we warmly recommend reading the generative AI design framework written by Vincent Koc. His "compass" focuses on six dimensions:
- Discovery, during which users discover GenAI through simple, engaging interactions.
- Assistance, which guides the experience without overriding user actions.
- Exploration, to encourage autonomy and creativity.
- Refinement, synonymous with personalization.
- Trust, by ensuring the quality and veracity of data produced by AI.
- Mastery, where users fully integrate AI into autonomous processes.
Excerpt from "GenAI Compass" - Source: Vincent Koc's article
The experts interviewed recommend introducing new AI features gradually, so that their use becomes habitual, thus referring to Raymond Loewy's notion of progressive design. A principle that the Doctrine teams applied when the @ was used as a shortcut in the chatbot: "Using @'s in a chatbot whether to invoke expertise or to ask a question about a specific document or entity is not yet "natural" for our customers, but we feel that it's an underlying trend that will gradually take hold in all chatbot UIs."
The question is also being asked at Cardiologs. Ingrid Pais de Oliveira talks about following a "less is more" philosophy in the workflow: "Every time we add a feature, we can't take the risk of changing the way doctors take the tool in hand in their own user journey. We can't afford onboarding. It has to be imperceptible".
Encouraging AI adoption and engagement
Once AI has been subtly integrated into the user journey, the next step is to encourage users to adopt it. Here again, we put ourselves in the user's shoes, but forgetting about any technological discourse, as Julien Laureau points out: "We really try to talk to the user in terms of benefits for them."
Keep in mind that digital experiences are rife with patterns, which facilitate this appropriation: for example, if I talk about a hamburger menu in Design review, nobody thinks I'm dreaming of fast food. AI-embedded features are no exception, and there are also a number of good practices, including:
- Giving users the option of disconnecting AI from their account: users, for reasons of privacy or lack of trust in technology, may prefer to keep control of the product they use.
- Highlight the options most used by the user when several are available. This is the principle behind the recommendations found on many catalog sites.
- Display the limitations of the model by letting the user take back control of the results, like the iPhone which asks the user to review the classification of his photos for example.
- Show that processing is underway, even if it means sometimes creating friction by simulating a longer process.
Maintaining the user's trust in AI
These practices bring to light a fundamental question of design with AI: how transparent should we be with users about the risks of error? Louis-Éric Maucout is emphatic: "With generative AI, no one can yet claim with certainty to provide a flawless answer. Business as usual is irresponsible, because the impact is too great for our customers". On the Doctrine chatbot, every source quoted is therefore transformed into a link directly in the answer, for one-click access. By removing as much friction as possible, Doctrine empowers its customers by encouraging them to check answers rather than rely on them blindly.
Translating uncertainty onto interfaces is necessary to gain user trust. In fact, data is not always as reliable and up-to-date as we'd like. In the medical field, this is literally a matter of survival. Ingrid Pais de Oliveira, Lead Designer at Cardiologs talks about "putting AI results in their proper place". She points out that "it's a result that's interpreted by an AI, never a diagnosis. We don't do the work for the user. At any moment, he can re-classify a signal."
It's through continuously observing and listening to users that the best ideas for AI features are born.
Another underlying question: should you openly mention that you're using AI in your product? The mention "AI" is sometimes displayed as a differentiating argument - we're thinking of the functionalities of business tools like Maze, Notion or Airtable - but it's not a generality. The term AI is not directly mentioned on the interfaces of Indy or Cardiologs, for example, although it is present on the latter's showcase site and slogan ("AI serving Cardiology").
🧗🏻♂️ Constantly adapting as a team
The technical nature of AI subjects can lead people to think that Designers have no say in the matter. However, the sooner they know the specifics of the model, the more relevant the path they will be able to propose. That's why after mentioning some good design practices, it's time to move on to some collaboration tips!
Strengthening collaboration with Data profiles
Let's start by deciphering the impacts of AI on the Tech <> Design <> Product partnership. Integrating AI presents new challenges for Designers. For example, data training can lengthen production release times, and this without any guarantee of results.
Data teams thus work daily on algorithms, revising model knowledge for reasons of security, confidentiality, data obsolescence or even to parameterize them for new needs. As an example, Cardiologs recently launched a pediatric version of its platform, which required technical adjustments (modifying the heart rate threshold) with impacts on user paths (identifying whether the file is that of a child or not). These specificities are fully integrated into the design process, as Ingrid Pais de Oliveira explains:"When we identify solutions, we will question the technical capabilities and regulatory adaptation of the solution. We need to know very quickly if what we're doing is legal, and if it's possible with AI."
It is therefore highly recommended to involve Data Scientists in all stages up to the creation of flows, during ideation workshops.
Testing AI with its users
However, users shouldn't remain on the sidelines. As we've seen, it's through continuous observation and listening to them that the best ideas for AI functionalities are born, i.e. those that truly meet a need. And what better way to train algorithms than with real user data? For reasons of confidentiality, however, this data may be deleted when the software goes into production.
.Let's close the loop, and also give users a say in the evaluation phase, when testing interfaces and tracking usage data. For their chatbot, the Doctrine teams thus studied the follow-up questions put to the chatbot, with the following focus: "Are these business questions that aim to dig deeper into a specific point of law, or is it a feature request, like getting more sources or summarizing the conversation?" If the second case is more recurrent, then the Designers make sure to respond to the user's request with a single click, by implementing a "More sources" or "Summarize the conversation" button. The latter are proposed as follow-up actions in the conversation.
The companies interviewed have taken the gamble - a successful one - of integrating AI right from the start. What if another key to success was to get Designers on board early on in this crazy adventure? Finding Product Designers with real expertise or an appetite for implementing AI is no easy task: for good reason, these functionalities, deemed technical, often remain in the hands of Product Managers and Data profiles.
Yet, Product Designers are the ones who can make the most of AI.
However, with their detailed knowledge of users' mental models, Product Designers think in terms of scripting and harmonious integration of AI into the user journey. Because they are attentive to cognitive overload and the clarity of the information delivered, they encourage users to explore AI according to their own needs, so that it is adopted with confidence. In fact, reassuring the user is indispensable in the design of artificial intelligence features. Anticipating biases, finding the right level of information and transparency to provide to the user must be major concerns to ensure responsible use of AI.
Finally for Product Designers, these are unprecedented times, as Louis-Éric Maucout testifies:"doing Product Design in these times doesn't happen often in a career. There are big bets to be made, it's a period of instability, but we're convinced we have big competitive advantages thanks to our strong Product culture and talented engineers."
Read our article on how AI is revolutionizing the job of Product Designer